
Optimization of Location Allocation of Web
Services Using A Modified Non-dominated
Sorting Genetic Algorithm
Boxiong Tan, Hui Ma, Mengjie Zhang
School of Engineering and Computer Science,
Victoria University of Wellington, New Zealand
{Boxiong.Tan, Hui.Ma, Mengjie.Zhang}@ecs.vuw.ac.nz
Abstract. In recent years, web services technology is becoming increas-
ingly popular because of the convenience, low cost and capacity to be
composed into high-level business processes. The service location-allocation
problem for a web service provider is critical and urgent, because some
factors such as network latency can make serious effect on the quality of
service (QoS). This paper presents a multi-objective optimization algo-
rithm based on NSGA-II to solve the service location-allocation problem.
A stimulated experiment is conducted using the WS-DREAM dataset.
The results are compared with a single objective genetic algorithm (GA).
It shows NSGA-II based algorithm can provide a set of best solutions
that outperforms genetic algorithm.
1 Introduction
Web Services are considered as self-contained, self-describing, modular appli-
cations that can be published, located, and invoked across the Web [25]. In recent
years, web services technology is becoming increasingly popular because of the
convenience, low cost [1] and capacity to be composed into high-level business
processes.
With the ever increasing number of functional similar web services being avail-
able on the Internet, the web service providers (WSPs) are trying to improve the
quality of service (QoS) to become competitive in the market. QoS, also known
as non-functional requirements to web services, is the degree to which a service
meets specified requirements or web service users’ needs [33], such as response
time, security and availability. Among numerous QoS measurements, service re-
sponse time is a critical factor for many real-time services, e.g. traffic service or
finance service. Service response time has two components: transmission time
(variable with message size) and network latency [18]. Study [17] shows that
network latency is a significant component of service response delay. Ignoring
network latency will underestimate response time by more than 80 percent [26],
since network latency is related to network topology as well as physical distance
[14]. To reduce the network latency WSPs need to allocate services location
where has the lower latency to the user center that access the services. User cen-
ter denotes a geometric location (e.g., a city) that is encompassed by a service
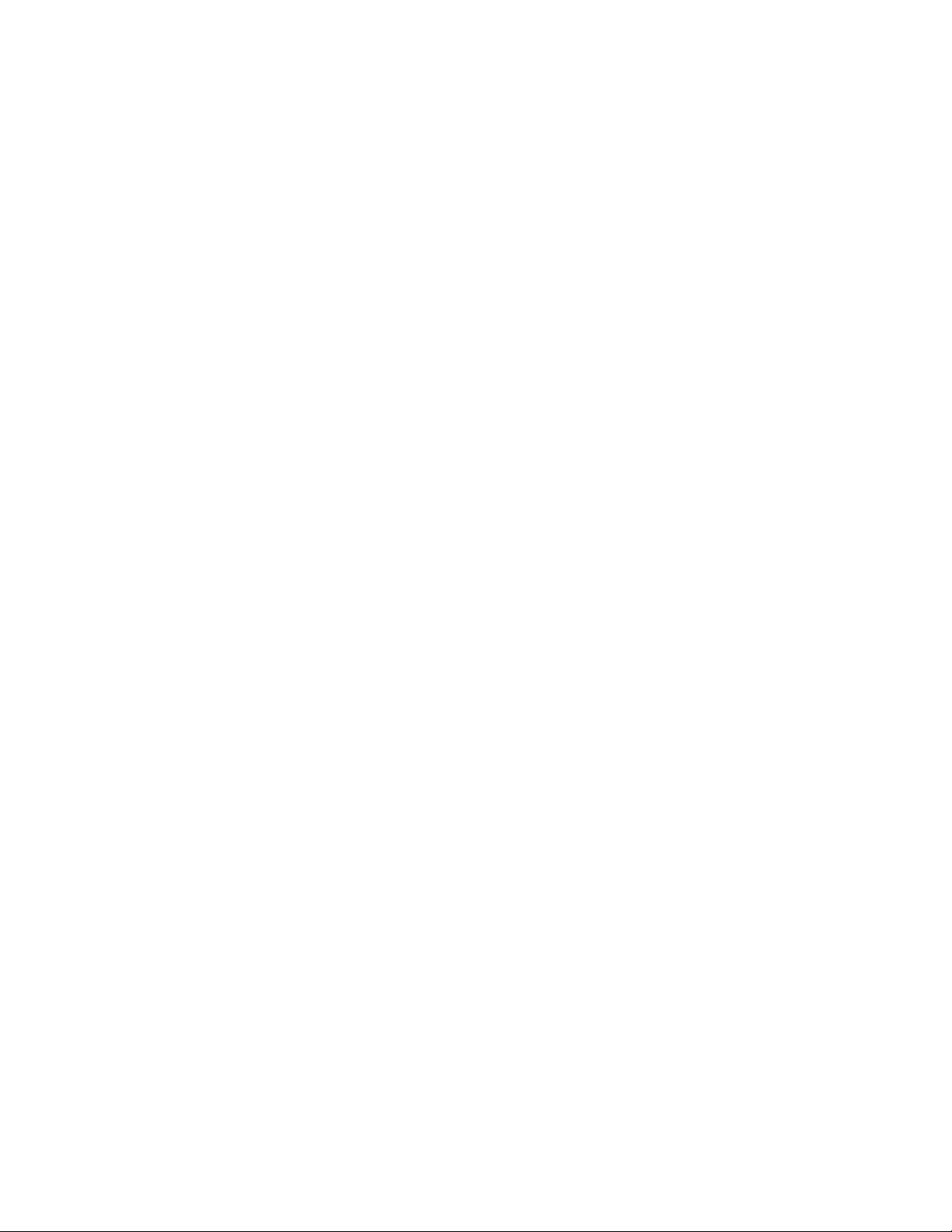
area. Service area, also known as trade area, is a region where web service users
concentrated at. There are many ways to identify service areas such as using
IP information or user generated location data [23]. Ideally, WSPs could deploy
their services to each user center in order to provide the best quality. However,
the more services deployed, the better the quality and the higher cost.
The Web service location-allocation problem is essentially a multi-objective
optimization problem [3], for which there are two conflict objectives, to provide
optimal QoS to web service users and to consume minimal deployment cost. This
problem can be classified as a multidimensional knapsack problem (MKP), there-
fore, it is considered NP-hard due to the fact that the combinatorial explosion
of the search space [27].
Very few researches have studied the service location-allocation problem and
most of the researchers treat this problem as a single objective problem. [1] [26]
try to solve the problem by using integer linear programming techniques. In par-
ticular, [26] solved this problem by employing greedy and linear relaxations of
Integer transpotation problem. However, integer programming (IP) is very effec-
tive for small-scale or mid-scale MKP but suffers from large memory requirement
for large-scale MKP [15]. Huang [12] proposed an enhanced genetic algorithm
(GA)-based approach, which make use of the integer scalarization technique to
solve this problem. This algorithm solves the problem with one objective and
one constraint. However there are some deficiencies in the integer scalarization
techniques [3]. Firstly, decision makers need to choose appropriate weights for
the objectives to retrieve a satisfactorily solution. Secondly, non-convex parts
of the Pareto set cannot be reached by minimizing convex combinations of the
object functions.
So far, to the best of our knowledge, there is no research has considered the
service location-allocation problem as a multi-objective problem. Therefore, this
paper we will treat service location-allocation problem as a multi-objective prob-
lem. Evolutionary multi-objective optimization (EMO) methodologies is ideal for
solving multi-objective optimization problems [7], since EMO works with a pop-
ulation of solutions and a simple EMO can be extended to maintain a diverse
set of solutions. With an emphasis for moving toward the true Pareto-optimal
region, an EMO can be used to find multiple Pareto-optimal solutions in one
single simulation run [19]. Among numerous EMO algorithms, Non-dominated
sorting GA (NSGA-II) [4], Strength Pareto Evolutionary Algorithm 2 (SPEA-2)
[5] have become standard approaches. Some schemes based on particle swarm
optimization (PSO) approaches are also proposed [10] [13]. NSGA-II is one of
the most widely used methods for generating the Pareto frontier, because it can
keep diversity without specifying any additional parameters [6]. In this paper,
we propose to use NSGA-II to solve the web service location-allocation problem,
which has two objectives, to minimize cost and deployment network latency.
The aim of this project is to propose a NSGA-II based approach to produce
a set of near optimal solutions of service location-allocation, so that cost and
overall network latency are close to minimum. Then, the WSPs could use the

algorithm which proposed by this paper, to select an optimal plan based on their
funds. The main objectives are:
– To model the web service location-allocation problem so that it can be tack-
led by NSGA-II.
– To develop a NSGA-II based approach to the web service location-allocation
problem.
– To evaluate our proposed approach using some existing datasets.
In Section 2 we introduce the background of NSGA-II and GA. In Section 3
we provide models of the service location allocation problems. Section 4 develops
a NSGA-II based algorithm. The experimental design and results evaluation are
shown in Section 6. Section 7 provides a brief summary.
2 Background
GA [21] is a powerful tool to solve combinatorial optimization problems. It is
an iterative procedure based on a constant-size population. In a GA, a popula-
tion of strings (called chromosomes or the genotype of the genome), which are
encoded as candidate solutions (called individuals, creatures, or phenotypes) to
an optimization problem, evolves towards better solutions. Each genome is as-
sociated with a fitness value based on a fitness function that indicates how close
it comes to meeting the overall specification, when compared to other genomes
in the population. The fitness value of an individual is also an indication of its
chances of survival and reproduction in the next generation. A typical genetic
algorithm requires a genetic representation of the solution domain and a fitness
function to evaluate the solution domain. Since a chromosome from the pop-
ulation represents a solution, when the algorithm starts, the whole population
moves like one group towards an optimal area so the GA searches from a popu-
lation of solutions rather than a single solution. Integer scalarization technique
[3] is used to solve multi-objective problems with GA. It predefines a weight for
each objective.
NSGA-II is a multi-objective algorithm based on GA. When used for problems
with only two objectives, NSGA-II performs relatively well in both convergence
and computing speed. However, NSGA-II has been criticized for its high compu-
tational cost and bad performance on applications with more than two objectives
[8].
NSGA-II permits a remarkable level of flexibility with regard to performance
assessment and design specification. NSGA-II assumes that every chromosome in
the population has two attributes: a non-domination rank in the population and
a local crowding distance in the population. The goal of NSGA-II is to converge
to the Pareto front as possible and with even spread of the solutions on the front
by controlling the two attributes.
The algorithm starts with a random initialization population. Once the pop-
ulation is sorted based on non-domination sorting, a rank is assigned to each
chromosome. Then, a parameter called crowding distance is calculated for each
individual. The crowding distance is a measure of how close an individual is to

its neighbors. A large average crowding distance will result in better diversity in
the population.
Parents are selected from the population by using tournament selection based
on the rank and the crowding distance. An individual is selected in the rank if it
is smaller than the other or if the crowding distance is greater than the other. The
selected population generates offsprings using crossover and mutation operators.
The population with the current population and current offsprings is sorted
again based on non-domination and only the best N individuals are selected,
where N is the population size. The selection is based on rank and the on crowd-
ing distance on the last front.
3 Problem Description and Modeling
In this section, we first describe the service location-allocation problem, then
we will present models for the services location allocation problem.
3.1 Problem Description and Assumptions
Web service location-allocation problem is to determine reasonable locations for
web services so that the deployment cost of WSP can be minimized while service
performance can be optimized. In this paper, to optimize service performance
we consider to minimize network latency.
The task of service location allocation has two objectives:
– To minimize the total cost of the services.
– To minimize the total network latency of the services.
To model the service location-allocation problem, we consider the following
assumptions:
Stakeholder Web Service Providers We consider the problem faced by a
WSP who has existing facilities but wishes to use the collected data to re-allocate
their services in order to maximum their profit.
The WSP must decide on facility locations from a finite set of possible loca-
tions. In order to make a decision, the WSP must first analyze the data collected
from current use of services. The collected data should include the records of in-
vocations from each unique IP address. Therefore, based on these data, the WSP
could summarize several customer demands concentrated on n discrete nodes [1],
namely user centers. We assume that the WSP has already done this step and a
list of user centers and candidate locations are given. Candidate location is the
geometric location that is suitable to deploy services. Candidate locations are
selected based on other criterions such as facilities or deployment cost. User cen-
ters and candidate locations can be overlapping, in fact, since web serivce users
receive best QoS services if the web serivces are deployed locally. Therefore, the
WSPs would like to choose user centers as candidate locations. In addition to
deciding locations to of the services, information about network latency between
user centers and candidate locations are needed.
The list below shows some critical information that should be providered by
the WSPs.
1. A list of user centers

2. A list of candidate locations
3. Service invocation frequency from user centers to services
4. Average network latency from user centers to candidate locations
5. Web service deployment cost for each candidate location
Worth nothing that service invocation frequency are changing over time. For
example, a service was popular in some regions may be unfrequented after a few
months. That’s the main reason for WSPs re-allocate their services. Network
latency highly depends on the network traffic and may be very different during
periods of a day. However, as long as there is no significant changes in the
network topology, the average network latency remain stable. Therefore, the
average network latency for a period of time should be representative.
These are the main input data that the decision making is dependent on. The
details of these input data and modeling are introduced in Section 3.2. Section
6 discussed the data that we used in the experiment.
Static deployment vs. Dynamic deployment As virtual machine technol-
ogy and infrastracture-as-a-service (IaaS) are becoming more and more popular.
Dynamic web service deployment become possible [20]. On the other hand, static
deployment is still the mainstream because of a majority of web serivce are de-
ployed on local infrastracture [11]. In this paper, we made an assumption that
WSPs periodicaly change the web service deployment since the user centers are
changing over time.
3.2 Model Formulation
To model service location-allocation problem, we need to make use of a set of
matrices, to present input information and output solutions.
For service location-allocation problem, we need information of service usage,
network latency, and service deployment cost to decide service location-allocation
so that the overall network latency can be minimized with minimal deployment
cost and within constraints. Assume a set of S = {s
1
, s
2
, ...s
s
, s
x
} services are re-
quested from a set of locations I = {i
1
, i
2
, ...i
i
, i
y
}. The service providers allocate
services to a set of candidate facility locations J = {j
1
, j
2
, ...j
j
, j
z
}.
In this paper, we will use the following matrices.
Matrices
L server network latency matrix L = {l
ij
}
A service location matrix A = {a
sj
}
F service invocation frequency matrix F = {f
is
}
C cost matrix C = {c
sj
}
R user response time matrix R = {r
is
}
service invocation frequency matrix, F = [f
is
], is used to record services invo-
cation frequencies from user centers, where f
is
is an integer that indicates the
number of invocations in a period of time from a user center to a service. For
example, f
13
= 85 denotes service s
1
is called 85 times in a predefined period of
time.