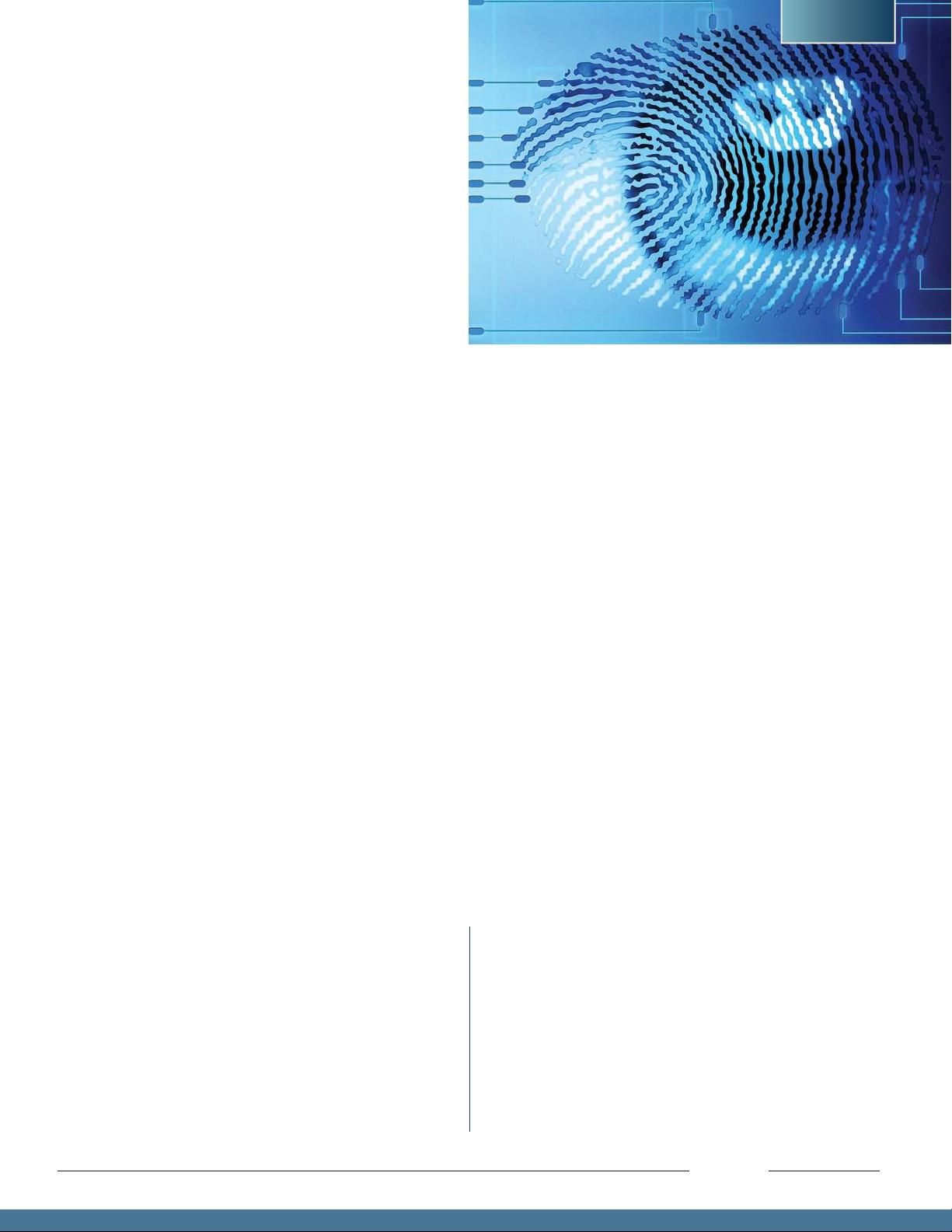
Abstract
A key contemporary trend emerging in big data science is the quantified self (QS)–individuals engaged in the self-
tracking of any kind of biological, physical, behavioral, or environmental inform ation as n = 1 individuals or in
groups. There are opportunities for big data scientists to develop new models to support QS data collection,
integration, and analysis, and also to lead in defining open-access database resources and privacy standards for
how personal data is used. Next-generation QS applications could include tools for rendering QS data meaningful
in behavior change, establishing baselines and variability in objective metrics, applying new kinds of pattern
recognition techniques, and aggregating multiple self-tracking data streams from wearable electronics, biosensors,
mobile phones, genomic data, and cloud-based services. The long-term vision of QS activity is that of a systemic
monitoring approach where an individual’s continuous personal information climate provides real-time perfor-
mance optimization suggestions. There are some potential limitations related to QS activity—barriers to wide-
spread adoption and a critique regarding scientific soundness—but these may be overcome. One interesting aspect
of QS activity is that it is fundamentally a quantitative and qualitative phenomenon since it includes both the
collection of objective metrics data and the subjective experience of the imp act of these data. Some of this dynamic
is being explored as the quantified self is becoming the qualified self in two new ways: by applying QS methods to
the tracking of qualitative phenomena such as mood, and by understanding that QS data collection is just the first
step in creating qualitative feedback loops for behavior change. In the long-term future, the quantified self may
become additionally transformed into the extended exoself as data quantification and self-tracking enable the
development of new sense capabilities that are not possible with ordinary senses. The individual body becomes a
more knowable, calculable, and administrable object through QS activity, and individuals have an increasingly
intimate relationship with data as it mediates the experience of reality.
Introduction
What is the quantified self?
The quantified self (QS) is any individual engaged in
the self-tracking of any kind of biological, physical, behav-
ioral, or environmental information. There is a proactive
stance toward obtaining information and acting on it. A
variety of areas may be tracked and analyzed, for example,
weight, energy level, mood, time usage, sleep quality, health,
cognitive performance, athletics, and learning strategies
(Table 1).
1
Health is an important but not exclusive focus,
where objectives may range from general tracking to pathology
resolution to physical and mental performance enhancement.
In some sense everyone is already a self-tracker since many
individuals measure something about themselves or have things
measured about them regularly, and also because humans have
innate curiosity, tinkering, and problem-solving capabilities.
One of the earliest recorded examples of quantified self-tracking
is that of Sanctorius of Padua, who studied energy expenditure
in living systems by tracking his weight versus food intake and
elimination for 30 years in the 16th century.
2
Likewise there is a
philosophical precedent for the quantified self as intellectuals
THE QUANTIFIED
SELF:
Fundamental Disruption in Big Data Science
and Biological Discovery
Melanie Swan
MS Futures Gro up, Palo Alto, California
REVIEW
DOI: 10.1089/big.2012.0002
MARY ANN LIEBERT, INC.
VOL. 1 NO. 2
JUNE 2013 BIG DATA
BD85

ranging from the Epicureans to Heidegger and Foucault have
been concerned with the ‘‘care of the self.’’ The terms ‘‘quanti-
fied self’’ and ‘‘self-tracker’’ are labels, contemporary formal-
izations belonging to the general progression in human history
of using measurement, science, and technology to bring order,
understanding, manipulation, and control to the natural world,
including the human body. While the concept of the quantified
self may have begun in n = 1 self-tracking at the individual level,
the term is quickly being extended to include other permuta-
tions like ‘‘group data’’—the idea of aggregated data from
multiple quantified selves as self-trackers share and work col-
laboratively with their data.
The Quantified Self in More Detail
The quantified self is starting to be a mainstream phenomenon
as 60% of U.S. adults are currently tracking their weight, diet,
or exercise routine, and 33% are monitoring other factors such
as blood sugar, blood pressure, headaches, or sleep patterns.
3,4
Further, 27% of U.S. Internet users track health data online,
5
9% have signed up for text message health alerts,
6
and there are
40,000 smartphone health applications available.
7
Diverse
publications such as the BBC,
8
Forbes,
9
and Vanity Fair
10
have
covered the quantified self movement, and it was a key theme
at CES 2013, a global consumer electronics trade show.
11
Commentators at a typical industry conference in 2012, Health
2.0, noted that more than 500 companies were making or
developing self-management tools, up 35% from the beginning
of the year, and that venture financing in the commensurate
period had risen 20%.
12
At the center of the quantified self
movement is, appropriately, the Quantified Self community,
which in October 2012 comprised 70 worldwide meetup
groups with 5,000 participants having attended 120 events
since the community formed in 2008 (event videos are avail-
able online at http://quantifiedself.com/). At the ‘‘show-and-
tell’’ meetings, self-trackers come together in an environment
of trust, sharing, and reciprocity to discuss projects, tools,
techniques, and experiences. There is a standard format in
which projects are presented in a simplified version of the
scientific method, answering three questions: ‘‘What did you
do?’’ ‘‘How did you do it?’’ and ‘‘What did you learn?’’ The
group’s third conference was held at Stanford University in
September 2012 with over 400 attendees. Other community
groups address related issues, for example Habit Design
(www.habitdesign.org), a U.S.-based national cooperative for
sharing best practices in developing sustainable daily habits via
behavior-change psychology and other mechanisms.
Exemplar quantified self projects
A variety of quantified self-tracking projects have been con-
ducted, and a few have been selected and described here to
give an overall sense of the diverse activity. One example is
design student Lauren Manning’s year of food visualization
(Fig. 1), where every type of food consumed was tracked over
a one-year period and visualized in different infographic
formats.
13
Another project is Tim McCormick’s Information
Diet, an investigation of media consumption and reading
practices in which he developed a mechanism for quantifying
the value of different infor mation inputs (e.g., Twitter feeds,
online news sites, blogs) to derive a prioritized information
stream for personal consumption.
14
A third example is Ro-
sane Oliveira’s multiyear investigation into diabetes and heart
disease risk, using her identical twin sister as a con trol, and
testing vegan dietary shifts and metabolism markers such as
insulin and glucose.
15
A fourth project nicely incorporating various elements of
quantified self-tracking, hardware hacking, quality-of-life
improvements, and serendipity is Nancy Dougherty’s smile-
triggered electromyogram (EMG) muscle sensor with an light
emitting diode (LED) headband display. The project is
Table 1. Quantified Self Tracking Categories and Variables
Physical activities: miles, steps, calories, repetitions, sets,
METs (metabolic equivalents)
Diet: calories consumed, carbs, fat, protein, specific
ingredients, glycemic index, satiety, portions, supplement
doses, tastiness, cost, location
Psychological states and traits: mood, happiness, irritation ,
emotions, anxiety, self-esteem, depression, confidence
Mental and cognitive states and traits: IQ, alertness, focus,
selective/sustained/divided attention, reaction, memory,
verbal fluency, patience, creativity, reasoning,
psychomotor vigilance
Environmental variables: location, architecture, weather,
noise, pollution, clutter, light, season
Situational variables: context, situation, gratification of
situation, time of day, day of week
Social variables: influence, trust, charism a, karma, current
role/status in the group or social network
Source: K. Augemberg.
1
(Reproduced with permission from
K. Augemberg)
FIG. 1. One year of food consumption visualization by Lauren
Manning.
THE QUANTIFIED SELF
Swan
86BD
BIG DATA JUNE 2013

designed to create unexpected moments of joy in human
interaction.
16
A fifth project of ongoing investigation has
been Robin Barooah’s personalized analysis of coffee con-
sumption, productivity, and meditation, with a finding that
concentration increased with the cessation of coffee drink-
ing.
17
Finally is Amy Robinson’s idea-tracking process in
which she e-mails ideas and inspirations to herself and later
visualizes them in Ge phi (an open-source graphing tool).
18
These projects demonstrate the range of topics, depth of
problem solving, and variety of methodologies characteristic
of QS projects. An additional indication of the tenor and
context of QS experimentation can be seen in exemplar
comments from the community’s 2012 conference (Table 2).
Tools for self-tracking and self-exper imentation
The range of tools used for QS tracking and experimentation
extends from the pen and paper of
manual tracking to spreadsheets,
mobile applications, and specialized
devices. Standard contemporary QS
devices include Fitbit pedometers,
myZeo sleep trackers, and Nike +
and Jawbone UP fitness trackers.
The Quantified Self web site listed
over 500 tools as of October 2012
(http://quantifiedself.com/guide/),
mostly concerning exercise, weight,
health, and goal achievement. Uni-
fied tracking for multiple activities is
available in mobile applications
such as Track and Share (www
.trackandshareapps.com) and Daily
Tracker (www.thedailytracker.com/).
19
Many QS solutions
pair the device with a web interface for data aggregation,
infographic display, and personal recommendations and ac-
tion plans. At present, the vast majority of QS tools do not
collect data automatically and require manual user data in-
put. A recent emergence in the community is tools created
explicitly for the rapid design and conduct of QS experi-
ments, including PACO, the Personal Analytics Companion
(https://quantifiedself.appspot.com/), and studycure (http://
studycure.com/).
Motivations for quantified self experimentation
Self-experimenters may have a wide range of motivations.
There is at least one study investigating self-tracking projects,
the DIYgenomi cs Knowledge Generation through Self-Ex-
perimentation Study (http://genomera.com/stud ies/knowl-
edge-generation-through-self-experimentation). The study
has found that the main reason individuals conducted QS
projects was to resolve or optimize a specific l ifestyle issue
such as sleep quality .
20
Another key
finding was that QS experimenters
often iterated through many differ-
ent solutions, and kinds of solutions,
before finding a final resolution
point. Some specific findings were
that poor sleep quality was the big-
gest factor that attributed to work
productivity for multiple individu-
als. For one individual, raising the
bed mattress solved the problem,
and for another, tracking and re-
ducing caffeine consumption. An-
other finding was that there was not
much introspection as to experi-
mental results and their meaning but
rather a pragmatic attitude toward having had a problem that
needed solving. A significant benefit of self-experimentation
projects is that the velocity of question asking and experiment
iterating can be much greater than with traditional methods.
At the meta-level, it is important to study the impact of the
practice of self-tracking. One reason is that health informa-
tion is itself an inte rvention.
21
Some studies have found that
there may be detrimental effects,
22
while others have docu-
mented the overall benefits of self-tracking to health and
wellness outcomes as well as the psychology of empowerment
and responsibility taking.
23–25
How the Quantified Self is Becoming an
Interesting Challenge for Big Data Science
Quantified self projects are becoming an interesting data
management and manipulation challenge for big data science
in the areas of data collection, integration, and analysis.
While quantified self data streams may not seem to conform
to the traditional concept and definition of big data—
‘‘data sets too large and complex to process with on-hand
database management tools’’ (http://en.wikipedia.org/wiki/
Big_data)—or connote examples like Walmart’s 1 million
Table 2. Quotable Quotes from the 2012
Quantified Self Conference
Can I query my shirt, or am I limited to consuming the
querying that comes packaged in my shirt?
Our mission as quantified selves is to discover our mission.
Data is the new oil.
The lean hardware movement becomes the lean heartware
movement.
Information wants to be linked.
We think more about our cats/dogs than we do our real
pets, our microbiome.
Information conveyance, not data visualization.
Quantified emotion and data sensation through haptics.
Display of numerical data and graphs are the interface.
Quantifying is the intermediary step.exosenses (haptics,
wearable electronic senses) is really what we want.
Perpetual data explosion.
The application of the metric distorts the data and the
experience.
‘‘ANOTHER FINDING WAS THAT
THERE WAS NOT MUCH
INTROSPECTION AS TO
EXPERIMENTAL RESULTS AND
THEIR MEANING BUT RATHER
A PRAGMATIC ATTITUDE
TOWARD HAVING HAD A
PROBLEM THAT NEEDED
SOLVING.’’
Swan
REVIEW
MARY ANN LIEBERT, INC.
VOL. 1 NO. 2
JUNE 2013 BIG DATA
BD87

transactions per hour being transmitted to databases that are
2.5 petabytes in size (http://wikibon.org/blog/big-data-statis-
tics/), the quantified self, and health and biology more gen-
erally, are becoming full-fledged big data problems in many
ways. First, individuals may not have the tools available on
local computing resources to store, query, and manipulate QS
data sets. Second, QS data sets are growing in size. Early QS
projects may have consisted of manageable data sets of man-
ually-tracked data (i.e., ‘‘small data’’). This is no longer the case
as much larger QS data sets are being generated. For example,
heart rate monitors, important for predictive cardiac risk
monitoring, take samples on the order of 250 times per second,
which generates 9 gigabytes of data per person per month.
Appropriate compression algorithms and a translation of the
raw data into aggregated data that would be more appropriate
for long-term storage have not yet been developed.
Another example is personal genomic data from ‘‘SNP chip’’
(i.e., single nucleotide polymorphism) companies like 23an-
dMe, Navigenics, and deCODEme. These files constitute 1–2%
of the human genome and typically have 1–1.2 million records,
which are unwieldy to load and query (especially when com-
paring multiple files) without specific data-management tools.
Whole human genome files are much larger than SNP files.
Vendors Illumina and Knome ship multi-terabyte-sized files to
the consumer in a virtually unusable format on a standalone
computer or zip drive. In the short-term, standard cloud-based
services for QS data storage, sharing, and manipulation would
be extremely useful. In the long-term, big data solutions are
needed to implement the vision of a systemic and continuous
approach to automated, unobtrusive data collection from
multiple sources that is processed into a stream of behavioral
insights and interventions. Making progress in the critical
contemporary challenge of preventive medicine–recognizing
early warning signs and eliminating conditions during the 80%
of their preclinical lifecycle—may likely require regular col-
lection on the order of a billion data points per person.
26
Specific big data science opportunities in data collection, in-
tegration, and analysis are discussed below in the sections data
collection, data integration, data analysis, and opportunities in
working with large data corpora.
Data collection: big health data streams
There is a need for big data scientists to facilitate the iden-
tification, collection, and storage of data streams related to
QS activity. Both traditional institutio nal health professionals
and QS individuals are starting to find themselves in a whole
new era of massively expanded data and have the attendant
challenge of employing these new data streams toward pa-
thology resolution and wellness outcomes. Big health data
streams can be grouped into three categories: traditional
medical data (personal and family health history, medication
history, lab reports, etc.), ‘‘omics’’ data (genomics, micro-
biomics, proteomics, metabolomics, etc.), and quantified-self
tracking data (Fig. 2).
27
A key shift is that due to the plum-
meting cost of sequencing and Internet-based data storage,
many of these data streams are now available directly to
consumers. In the omics category of health data streams, as of
January 2013 genomic profiling was available for $99 from
23andMe (sequencing 1 million of the most-researched
SNPs) and microbiomic profiling was available for $79 from
uBiome (www.indiegogo.com/ubiome) and $99 from the
American Gut Project (www.indiegogo.com/americangut). A
broad consumer application of integrated omics data streams
is not yet available, as institutional projects
28,29
are them-
selves in early stages, but could quickly emerge from acade-
mia through consumer proteomics services such as Talking20
(the body’s 20 amino acids) who offers $5 home blood-test
cards for a multi-item pane l (e.g., vitamins, steroids, and
cholesterol).
30
Data integration
A key challenge in QS projects and the realization of pre-
ventive medicine more generally is integrating big health data
streams, especially blending genomic and environmental
data. As U.S. National Institutes of Health director Francis
Collins remarked in 2010, ‘‘Genetics loads the gun and en-
vironment pulls the trigger.’’
31
It is a general heuristic for
common disease conditions like cancer and heart disease that
genetics have a one-third contribution to outcome and en-
vironment two-thirds.
32
There are some notable examples of
QS projects involving the integration of multiple big health
data streams. Self trackers typically obtain underlying geno-
mic and microbiomic profiling and review this information
together with blood tests and proteomic tests to determine
baseline levels and variability for a diversity of markers
and then experiment with different interventions for opti-
mized health and pathology reduction. Some examples
of these kinds of QS data integration projects include
DIYgenomics studies,
33
Leroy Hood’s 4P medicine (predictive,
FIG. 2. Big health data streams are becoming increasingly
consumer-available.
THE QUANTIFIED SELF
Swan
88BD
BIG DATA JUNE 2013

personalized, preventive, and participatory),
26
David Duncan’s
Experimental Man project,
34
Larry Smarr’s Crohn’s disease
tracking microbiomic sequencing and lactoferrin analysis
project,
35
and Steven Fowkes’s Thyroid Hormone Testing
project.
36
Studies may be conducted individually (n = 1), in
groups (aggregations of n = 1 individuals), or in systems (e.g.,
families, athletic teams, or workplace groups). For group
studies, crowdsourced research collaborations, health social
networks, and mobile applications are allowing studies to be
conducted at new levels of scale and specificity, for example,
having thousands of participants as opposed to dozens or
hundreds.
37,38
The ability to aggregate dozens of QS data streams to look for
correlations is being developed by projects such as Singly,
Fluxstream, Bodytrack, Sympho.Me, Sen.se, Cosm, and the
Health Graph API.
39
Figure 3 shows a ‘‘mulitviz’’ display
from Sen.se that plots coffee consumption, social interaction,
and mood to find apparent linkage between social interaction
and mood, although correlation is not necessarily causa-
tion.
40
The aggregation of multiple
data streams could be a preliminary
step toward two-way communica-
tion in big data QS applications that
offer real-time inter ventional sug-
gestions based on insights from
multifactor sensor input processing.
This kind of functionality could be
extended to the development of
flexible services that respond in real-
time to demand at not just the in-
dividual level but also the commu-
nity level. A concrete example could
be using the timing, type, and cy-
clicality of 4 million purchase transactions that occurred
during Easter week in Spain (http://senseable.mit.edu/bbva/)
to design flexible bank, gas station, and store hours, and
purchase recommendation services that respond in real-time
to community demand.
Data analysis
Following data collect ion and integration, the next step is
data analysis. A classic big data science problem is extracting
signal from noise. The objective of many QS projects is sifting
through large sets of collected data to find the exception that
is the sign of a shift in pattern or an early warning signal.
Ultimately, 99% of the data may be useless and easily dis-
carded. However, since continuous monitoring and QS
sensing is a new area and use cases have not been defined and
formalized, much of the data must be stored for character-
ization, investigation, and validation. A high-profile use case
is heart failure, where there is typically a two-week prevention
window before a cardiac event during which heart rate var-
iability may be predictive of pathology development. Trans-
lating heart rate data sampled at 250 times per second into
early warnings and intervention is an unres olved challenge.
One thing that could help is the invention of a new genera-
tion of data compression algorithms that could allow
searching and pattern-finding within compressed files. Si-
milar to the challenge of producing meaningful signals from
heart-rate variability data is the ex-
ample of galvanic skin response
(GSR). Here too, data metrics that
are sampled at many times per sec-
ond have been available for decades,
but the information has been too
noisy to produce useful signals cor-
related with external stimulus and
behavior. It is only through the ap-
plication of innovations in multiple
areas—hardware design, wearable
biosensors, signal processing, and
big data methods—that GSR infor-
mation is starting to become more
useful.
41
Analyzing multiple QS data streams in real-time (for
example, heart-r ate variability, galvanic skin response, tem-
perature, movement, and EEG activity) may likely be re-
quired for accurate assessment and intervention regarding
biophysical state.
FIG. 3. Seeking correlations: multiviz data stream infographing available on the Sen.se Platform.
40
(Reproduced with permission from
Sen.se)
‘‘THE OBJECTIVE OF MANY QS
PROJECTS IS SIFTING
THROUGH LARGE SETS OF
COLLECTED DATA TO FIND
THE EXCEPTION THAT IS THE
SIGN OF A SHIFT IN PATTERN
OR AN EARLY WARNING
SIGNAL.’’
Swan
REVIEW
MARY ANN LIEBERT, INC.
VOL. 1 NO. 2
JUNE 2013 BIG DATA
BD89