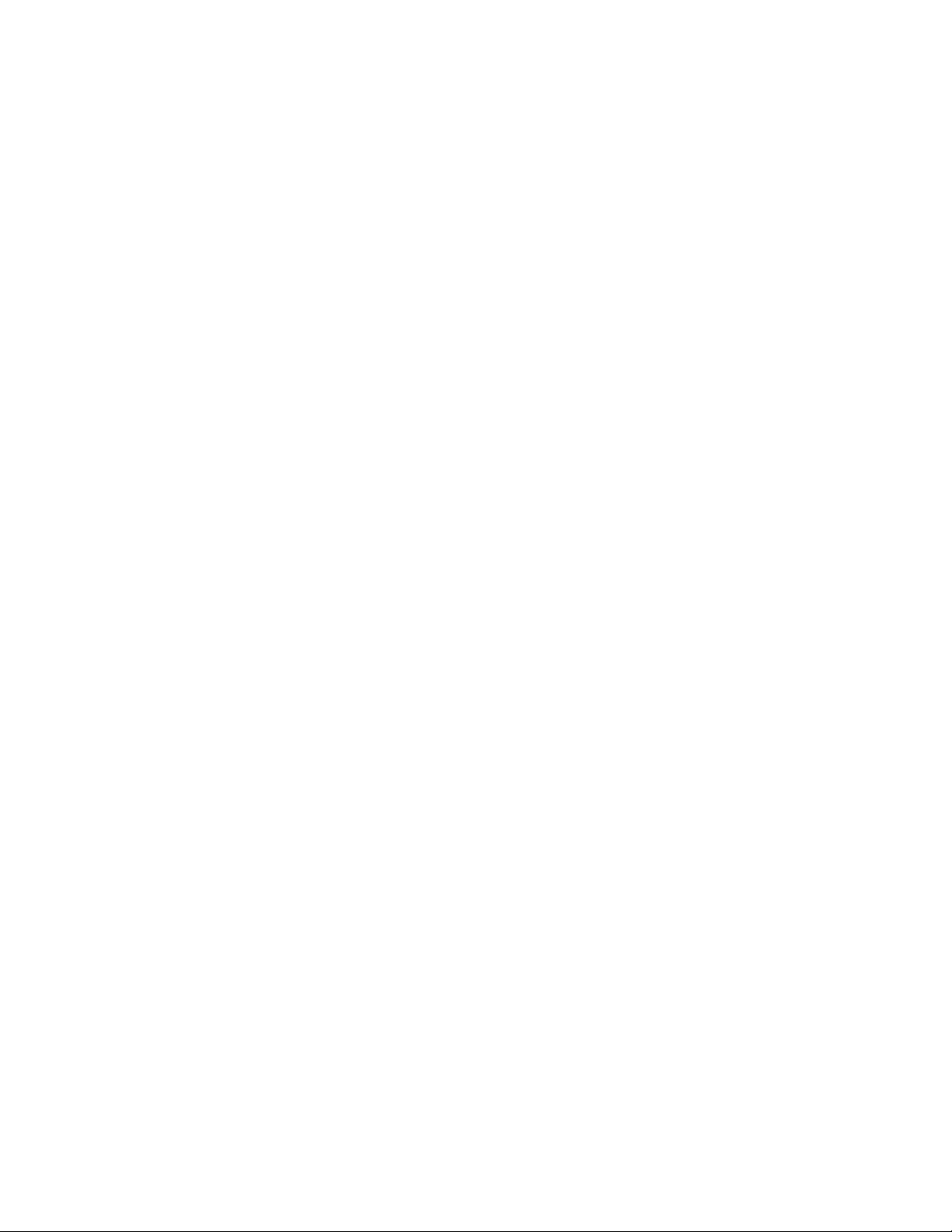
A
Relay-Assisted Distributed Source Coding
Problem
Prakash Ishwar
Dept. Electrical and Computer Engineering
Boston University
Boston, MA 02215, USA
Email: pi@bu.edu
S. Sandeep Pradhan
Dept. Electrical Engineering and Computer Science
University of Michigan
Ann Arbor, MI 48109, USA
Email: pradhanv@eecs.umich.edu
Abstract— A relay-assisted distributed source coding problem
with three statistically correlated sources is formulated and
studied. Natural lower bounds for the rates are presented. The
lower bounds are shown to be achievable for three special classes
of joint source distributions. The achievable coding strategies for
the three special classes are observed to have markedly different
characteristics. A special class for which the lower bounds are not
achievable is presented. A single unified coding strategy which
subsumes all four special classes is derived.
I. I
Consider the following distributed source coding problem
which is illustrated in Fig. 1. n samples X
n
:= (X
1
, . . . , X
n
)
of an information source available at location-a need to be
reproduced at location-c with high probability. The message
from location-a, of bit rate R
a
bits per source sample, is
relayed through an intermediate location-b which has access
to n samples Y
n
of a second information source which are
statistically correlated to X
n
. The message from location-b to
location-c is of bit rate R
b
bits per source sample. Location-c
has access to n samples Z
n
of a third information source which
are correlated to X
n
and Y
n
. The reproduction at location-c is
b
X
n
. The goal is to characterize the set of minimal rate pairs
(R
a
, R
b
) for which P(X
n
,
b
X
n
) ↓ 0 as n ↑ ∞ (the rate region).
The problem just described belongs to the general class of
source network problems [1, Chapter 3] (also called distributed
source coding problems) which have been studied in the Infor-
mation Theory literature in the 70’s and 80’s. Beginning the
late 90’s, the construction of practical distributed source codes
for emerging wireless sensor network applications [2] received
much attention and renewed interest in the study of general
source network problems. For instance, the three sources in
Fig. 1 can represent the correlated observation samples of
three physically separated, radio-equipped, sensing devices
which are measuring some physical quantities of interest in the
vicinity of their respective locations. In some situations, the
device at location-c may need to learn about the observations
at location-a but is uninterested in the observations of an
intermediate location-b whose connectivity with both location-
a and location-c is orders of magnitude stronger than that
between location-a and location-c. Depending on the relative
strengths of the connections between location-a and location-b
and between location-b and location-c, it may be necessary to
use codes of different rates on these links to achieve the desired
objective of reproducing X
n
at location-c. This motivates the
characterization of the entire rate region of minimal rate pairs
(R
a
, R
b
) for which P(X
n
,
b
X
n
) can be driven down to zero as
n ↑ ∞. More recently, the study of general source networks
has also benefited from insights which are emerging from the
related area of network coding [3].
In the terminology of [1, p. 246], the source network of
Fig. 1 has three input vertices corresponding to the three
sources, one output vertex corresponding to the decoder at
location-c, and two intermediate vertices corresponding to the
encoders at locations a and b. The depth of this network which
is the length of the longest path from an input to an output is
3. The structure of this network is significantly different from
that of several related source network problems of depth 2
studied in the 70’s and 80’s whose rate regions are fairly well
understood (see [4], [1, Chapter 3], and references therein). In
more recent terminology, the specific source network of Fig. 1
would be the simplest example of the so-called single-demand
line source networks (see for example [5], [6]).
Despite their apparent simplicity, the general rate regions of
line source networks are not well understood except in very
special cases when the joint probability distribution of the
sources and/or the fidelity criteria for source reproductions
have some special properties. For instance, inner and (non-
matching) outer bounds for the rate-distortion region for
a slightly more general class of tree source networks are
derived in [5]. However, it is assumed that there exists an
underlying hidden source conditioned on which all the source
observations become independent. Moreover, all locations
need to reproduce the hidden source to within some fidelity
as measured by a single-letter distortion function. A recent
closely related work [7] studies the relay-assisted distributed
source coding problem of Fig. 1 but when X
n
–Z
n
–Y
n
forms
a Markov chain (degraded side information) and when X
n
needs to be reproduced at location-b and location-c only to
within expected distortion levels as measured by associated
single-letter fidelity criteria. Inner and (non matching) outer
bounds for the rate-distortion region are derived and some
special cases are discussed. In the context of asymptotically
lossless reconstruction of X
n
at (only) location-c, the condition
X
n
–Z
n
–Y
n
corresponds to the special case 2 in Section III-B.
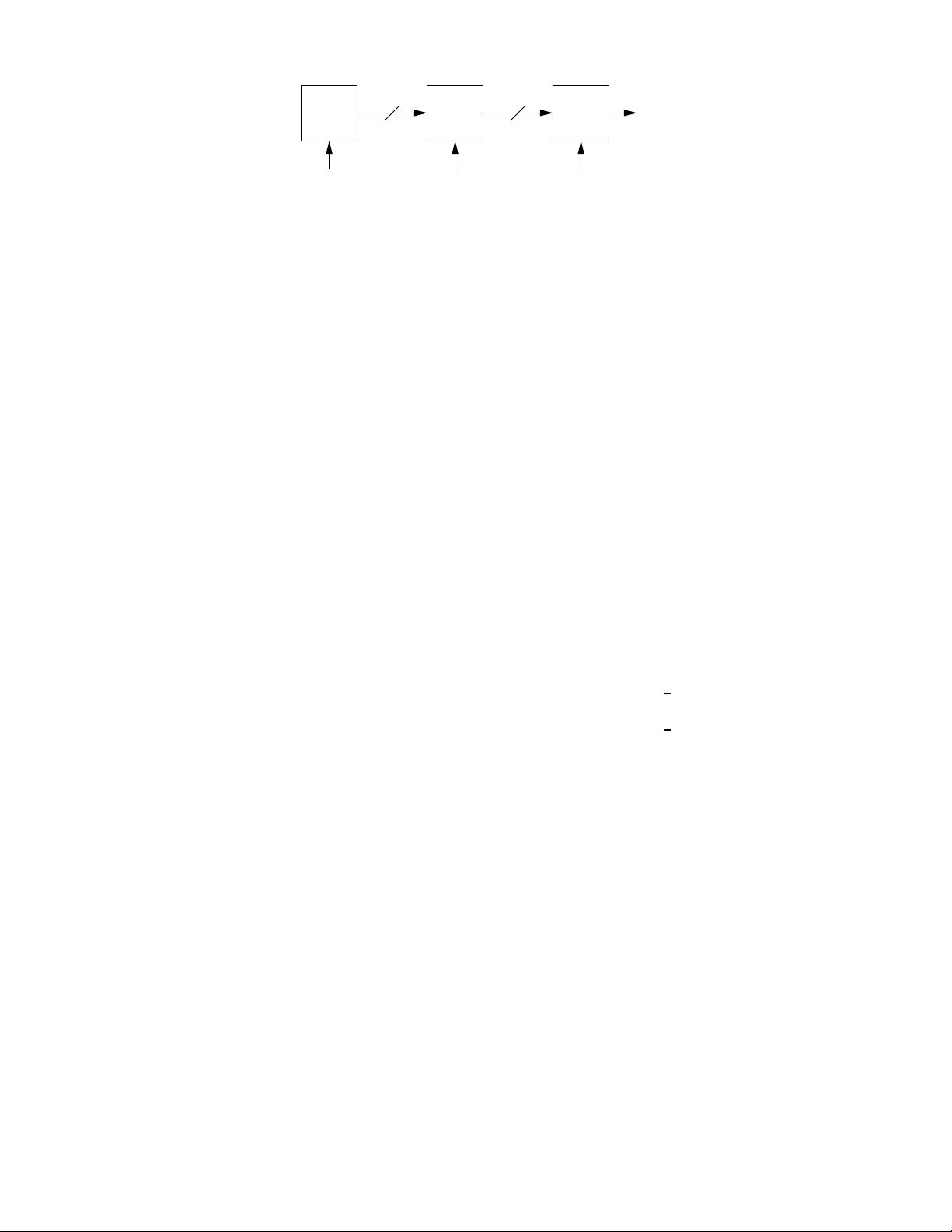
R
b
a b c
X
n
Y
n
Z
n
b
X
n
R
a
Fig.
1. Relay-assisted distributed source coding.
Other related works include [8], [9].
As discussed in what follows, the study of even the sim-
plest line source network with three sources (Fig. 1) offers
new insights for efficient information processing and coding
in source networks. The presence of an intermediate relay
with correlated side information Y
n
makes the problem both
interesting and challenging in a number of ways. Perhaps the
two most striking features of this problem are the following.
(i) It is not necessary for the relay to reproduce the source
X
n
.
1
Hence, the relay need not “fully decode” the message
from location-a. (ii) The presence of correlated Y
n
at the relay
should be helpful but it is not necessary that Y
n
be reproduced
at location-c for X
n
to be reproduced at location-c. Thus we see
that there is a very interesting set of interactions and tradeoffs
which come into play in this problem. These considerations
also give rise to a number of distinct coding strategies for the
relay. For instance, the relay can simply forward the message
from location-a to location-c without any processing, it can
encode some of its own observations and convey it to location-
c, or it can process the message from location-a using Y
n
to
decode an intermediate description of X
n
and then jointly re-
encode the intermediate description together with a part of Y
n
and send it to location-c, etc.
The problem is mathematically formulated in Section II.
Section III derives a natural outer bound for the rate region
and discusses three special source correlation structures for
which the outer bound is tight. The study of these three special
cases is rewarding because it exposes three coding strategies
of significantly different flavors. An interesting special case
for which the outer bound is not tight is also presented. The
distinctiveness of the three coding strategies motivates the
search for a single unified coding strategy which subsumes
all the previously discussed strategies as special cases. This
is accomplished by Theorem 1 in Section IV. Concluding
remarks are made Section V.
Notation: For n ∈ N, a
n
:= (a
1
, . . . , a
n
). Kronecker delta
δ
uv
: δ
uv
= 1 if u = v and is zero otherwise.
II. S P
Let ((X
i
, Y
i
, Z
i
))
n
i=1
, n ∈ N, be a three-component discrete-
time, memoryless, stationary source, that is, for i = 1, 2, 3, . . . ,
(X
i
, Y
i
, Z
i
) ∼ i.i.d. p
X,Y,Z
(x, y, z) with (x, y, z) ∈ X × Y × Z.
It is assumed that max{|X|, |Y|, |Z|} < ∞. X
n
is observed at
1
If X
n
is also required to be reproduced at location-b then the problem
is much easier and the rate region is immediate (cf. remark at the end of
Section II). Thus the interesting problem is when X
n
must be reproduced at
only location-c.
location-a, Y
n
at location-b, and Z
n
at location-c. The goal
is to reproduce X
n
at location-c. The constraint is that the
message from location-a has to pass through the intermediate
location-b. The problem is illustrated and summarized in Fig. 1
Definition 1: A relay-assisted distributed source code with
parameters (n, m
a
, m
b
) is the triple (e
a
, e
b
, g
c
):
e
a
: X
n
−→ {1, . . . , m
a
}, e
b
: {1, . . . , m
a
}×Y
n
−→ {1, . . . , m
b
},
g
c
: {1, . . . , m
b
} × Z
n
−→ X
n
.
Here, n is the blocklength, e
a
will be called the source
encoder, e
b
will be called the relay encoder, and g
c
will
be called the source decoder. The quantities (1/n) log
2
(m
a
)
and (1/n) log
2
(m
b
) will be called the source and relay
block-coding rates (in bits per sample) respectively.
Definition 2: A rate-pair (R
a
, R
b
) is said to be operationally
admissible for relay-assisted distributed source coding if, for
every > 0, there exists an N
∈ N, such that, for all n ≥
N
, there exists a relay-assisted distributed source code with
parameters (n, m
a
, m
b
) satisfying
1
n
log
2
(m
a
) ≤ R
a
+
, (2.1)
1
n
log
2
(m
b
) ≤ R
b
+
, (2.2)
P(g
c
(e
b
(e
a
(X
n
), Y
n
), Z
n
) , X
n
) ≤ . (2.3)
Let W
a
:= e
a
(X
n
), W
b
:= e
b
(W
a
, Y
n
),
b
X
n
:= g
c
(W
b
, Z
n
), and
P
e
:= P(
b
X
n
, X
n
).
Definition 3: The operationally admissible rate region for
relay-assisted distributed source coding, R
op
, is the set of
all operationally admissible rate-pairs for relay-assisted
distributed source coding.
It can be verified from the above definitions that R
op
is a
closed convex subset of [0, ∞)
2
which contains [log
2
|X|, ∞)
2
.
Problem statement: Characterize R
op
in terms of computable
single-letter [1] information quantities.
Remark: The problem of characterizing the rate region be-
comes considerably easier if X
n
has to be reproduced at both
location-b and location-c with an error probability which tends
to zero as n tends to infinity. The rate region in this case
is given by R
a
≥ H(X|Y), R
b
≥ H(X|Z): For any arbitrarily

small
positive real number and all n sufficiently large, X
n
can be encoded at location-a at the rate H(X|Y) bits per
sample, and decoded at location-b with a probability which
can be made smaller than . This follows from the coding
theorem due to Slepian and Wolf [10] with X
n
as the source
of information to be encoded at location-a and Y
n
as the side-
information available to a decoder at location-b. The Slepian-
Wolf coding theorem applied a second time with X
n
as the
source of information available at location-b and Z
n
as the
side-information available to a decoder at location-c shows
that, for any arbitrarily small positive real number and all
n sufficiently large, X
n
can be re-encoded at location-b at the
rate H(X|Z) bits per sample, and decoded at location-c with
a probability which can be made smaller . The converse to
the Slepian-Wolf coding theorem proves that the lower bound
R
a
≥ H(X|Y) cannot be improved. The cutset bound (3.5) in
Section III-A proves that the lower bound R
b
≥ H(X|Z) cannot
be improved. Thus the rate-region is fully characterized.
III. S C
A. The cutset bounds
Proposition 1: If a rate-pair (R
a
, R
b
) is operationally admis-
sible for relay-assisted distributed source coding then
R
a
≥ H(X|Y, Z), (3.4)
R
b
≥ H(X|Z) (3.5)
where (X, Y, Z) ∼ p
X,Y,Z
(x, y, z) and H(X|Z), H(X|Y, Z), are
conditional entropies [10] in bits.
Proof: (R
a
, R
b
) ∈ R
op
⇒ ∀ > 0, ∃N
∈ N : ∀n ≥ N
, there
exists a relay-assisted distributed source code with parameters
(n, m
a
, m
b
) satisfying conditions (2.1), (2.2), and (2.3). By
Fano’s inequality [10],
H(X
n
|
b
X
n
) ≤ 1 + nP
e
log
2
|X| ≤ 1 + n log
2
|X|. (3.6)
For all > 0 and all n ≥ N
, we have the following information
inequalities
R
a
(a)
≥
1
n
log
2
m
a
−
(b)
≥
1
n
H(W
a
) −
(c)
≥
1
n
H(W
a
|Y
n
, Z
n
) −
(d)
=
1
n
I(W
a
; X
n
|Y
n
, Z
n
) −
=
1
n
(
H(X
n
|Y
n
, Z
n
) − H(X
n
|Y
n
, Z
n
, W
a
)
)
−
(e)
= H(X|Y, Z) −
1
n
H(X
n
|Y
n
, Z
n
, W
a
, W
b
,
b
X
n
) −
( f )
≥ H(X|Y, Z) −
1
n
H(X
n
|
b
X
n
) −
(g)
≥ H(X|Y, Z) −
1
n
−
log
2
|X| + 1
where
(a) is due to (2.1), (b) is because W
a
takes no more than
m
a
distinct values, (c) because conditioning does not increase
entropy, (d) because W
a
is a deterministic function of X
n
, (e)
is because (X
i
, Y
i
, Z
i
) ∼ i.i.d. p
X,Y,Z
(x, y, z) and because W
b
and
b
X
n
are respectively functions of (W
a
, Y
n
) and (W
b
, Z
n
), ( f ) is
because unconditioning does not decrease entropy, and (g) is
due to Fano’s inequality (3.6). Since the last inequality holds
for all > 0 and all n ≥ N
,
R
a
≥ H(X|Y, Z).
In a similar manner, for all > 0 and all n ≥ N
, we have the
following information inequalities
R
b
(a)
≥
1
n
log
2
m
b
−
(b)
≥
1
n
H(W
b
) −
(c)
≥
1
n
H(W
b
|Z
n
) −
≥
1
n
I(W
b
; X
n
|Z
n
) −
=
1
n
(
H(X
n
|Z
n
) − H(X
n
|Z
n
, W
b
)
)
−
(d)
= H(X|Z) −
1
n
H(X
n
|Z
n
, W
b
,
b
X
n
) −
(e)
≥ H(X|Z) −
1
n
H(X
n
|
b
X
n
) −
( f )
≥ H(X|Z) −
1
n
−
log
2
|X| + 1
where
(a) is due to (2.2), (b) is because W
b
takes no more than
m
b
distinct values, (c) because conditioning does not increase
entropy, (d) is because (X
i
, Z
i
) ∼ i.i.d. p
X,Z
(x, z) and because
b
X
n
is a deterministic function of (W
b
, Z
n
), (e) is because
unconditioning does not decrease entropy, and ( f ) is due to
Fano’s inequality (3.6). Since the last inequality holds for all
> 0 and all n ≥ N
,
R
b
≥ H(X|Z).
B.
Special cases in which the cutset bounds are tight
Case-1: X–Y–Z is a Markov chain. In this case,
H(X|Y, Z) = H(X|Y). Thus for any arbitrarily small
positive real number and all n sufficiently large, X
n
can be encoded at location-a at the rate H(X|Y, Z) bits
per sample, and decoded at location-b with a probability
which can be made smaller than . This follows from
the Slepian-Wolf coding theorem with X
n
as the source
of information to be encoded at location-a and Y
n
as
the side-information available to a decoder at location-b.
The Slepian-Wolf coding theorem applied a second
time with X
n
as the source of information available at
location-b and Z
n
as the side-information available to
a decoder at location-c shows that, for any arbitrarily
small positive real number and all n sufficiently

lar
ge, X
n
can be re-encoded at location-b at the rate
H(X|Z) bits per sample, and decoded at location-c with
a probability which can be made smaller . We shall call
this relay-assisted distributed source coding strategy as
relay decode and re-encode.
Case-2: X–Z–Y is a Markov chain. In this case,
H(X|Y, Z) = H(X|Z). The Slepian-Wolf coding theorem
with X
n
as the source of information available at
location-a and Z
n
as the side-information available to
a decoder at location-c shows that, for any arbitrarily
small positive real number and all n sufficiently large,
X
n
can be encoded at location-a at the rate H(X|Y, Z) bits
per sample, and decoded at location-c with a probability
which can be made smaller than . The operation at the
intermediate location-b is to simply forward the message
from location-a to location-c without any processing. We
shall call this relay-assisted distributed source coding
strategy as relay forward.
Case-3: H(Y|X, Z) = 0, that is, for all tuples (x, z) ∈ X×Z
for which p
X,Z
(x, z) > 0, y is a deterministic function
of (x, z). In this case, H(X|Z) = H(Y|Z) + H(X|Y, Z)
which is seen be expanding H(X, Y|Z) in two different
ways by the chain rule for conditional entropy [10]. The
Slepian-Wolf coding theorem with Y
n
as the source of
information available at location-b and Z
n
as the side-
information available to a decoder at location-c shows
that, for any arbitrarily small positive real number and
all n sufficiently large, Y
n
can be encoded at location-b at
the rate H(Y|Z) bits per sample, and decoded at location-
c with a probability which can be made smaller than
. The Slepian-Wolf coding theorem applied a second
time with X
n
as the source of information available at
location-a and (Y
n
, Z
n
) as the side-information available
to a decoder at location-c shows that, for any arbitrarily
small positive real number and all n sufficiently large,
X
n
can be encoded at location-a at the rate H(X|Y, Z) bits
per sample, and decoded at location-c with a probability
which can be made smaller than . The operation at the
intermediate location-b is to (i) encode its observations to
be reproduced at location-c and (ii) forward the message
from location-a to location-c without any processing. We
shall call this relay-assisted distributed source coding
strategy as relay encode and forward.
C. A case in which the cutset bounds are not tight
Case-4: X = Y = Z = {0, 1}, p
Y,Z
(y, z) = 0.5(1 −
p)δ
yz
+ 0.5p(1 − δ
yz
), p ∈ (0, 1), and X = Y ∧ Z where
Y ∧ Z is the Boolean AND function. According to the
cutset bounds, R
a
≥ H(X|Y, Z) = 0 and R
b
≥ H(X|Z) =
0.5H(Y ∧ Z|Z = 1) = 0.5H(Y|Z = 1) = 0.5h
2
(p) where
h
2
(α) := −α log
2
(α) − (1 − α) log
2
(1 − α), α ∈ [0, 1] is
the binary entropy function [10]. If R
a
= 0 then it can
be argued that the problem is equivalent to a depth-2
network source coding problem with sources Y
n
and Z
n
available at location-b and location-c respectively and the
goal being to reproduce the sample-wise Boolean AND
function of Y
n
and Z
n
at location-c with a probability
which tends to one as n tends to infinity. This latter
problem has been studied by Yamamoto [11] and by
Han and Kobayashi [12]. Lemma 1 in [12] applied to
the current problem proves that R
b
≥ H(Y|Z) = h
2
(p) >
0.5h
2
(p). Thus the cutset bounds are not tight for general
p
X,Y,Z
.
IV. A G I R R
Theorem 1: (Single coding strategy covering all cases) Let
(X, Y, Z, U, V) be random variables taking values in X × Y ×
Z × U × V, where U and V are finite alphabets. For all
(x, y, z, u, v) ∈ X × Y × Z × U × V, let
p
X,Y,Z,U,V
(x, y, z, u, v) = p
X,Y,Z
(x, y, z) · p
U|X
(u|x) · p
V|U,Y
(v|u, y),
where p
U|X
(·|·) and p
V|U,Y
(·|·) are conditional pmfs, that is,
the auxiliary random variables U, V satisfy the following two
Markov chains: U–X–(Y, Z) and V–(U, Y)–(X, Z). Then all
rate-pairs (R
a
, R
b
) satisfying
R
a
≥ I(X; U|Y) + H(X|V, Z) (4.7)
R
b
≥ I(Y, U; V|Z) + H(X|V, Z) (4.8)
are operationally admissible for relay-assisted distributed
source coding and cover all the four previously discussed
special cases.
Proof-sketch: We first show that all the four previously dis-
cussed special cases are covered by Theorem 1. For Case-1,
set U = V = X and U = V = X, for Case-2, set |U| = |V| = 1,
for Cases 3 and 4, set |U| = 1, V = Y, and V = Y.
We now sketch the proof that all rate-pairs (R
a
, R
b
) satis-
fying (4.7) and (4.8) are operationally admissible for relay-
assisted distributed source coding. The proof sketch follows
well known random coding and random binning arguments in
[1], [10], [13].
Let (X, Y, Z, U, V) be random variables taking values in X ×
Y × Z × U × V with the joint pmf p
X,Y,Z,U,V
(x, y, z, u, v) =
p
X,Y,Z
(x, y, z)·p
U|X
(u|x)·p
V|U,Y
(v|u, y) as stated in Theorem 1. Let
X := X
n
, Y := Y
n
, Z := Z
n
, > 0, and C
x
:= (x(1), . . . , x(|X|
n
))
be an ordering of the set of all sourcewords X
n
.
Random codebook generation for location-a: Generate m
0
u
i.i.d. codewords U( j) := U
n
( j), j = 1, . . . , m
0
u
, of block-
length n, according to the product pmf p
U
(u) :=
Q
n
i=1
p
U
(u
i
),
independent of (X, Y, Z). The tuple of codewords C
u
:=
(U(1), . . . , U(m
0
u
)) is available at locations a and b. Generate
m
0
u
i.i.d. bin-indices A
j
, j = 1, . . . , m
0
u
, according to the
uniform pmf over the set {1, . . . , m
u
}, independent of (X, Y, Z)
and {U( j), j = 1, . . . , m
0
u
}. The tuple of bin-indices A :=
(A
1
, . . . , A
m
0
u
) is available at locations a and b.
The interpretation is that for j = 1, . . . , m
0
u
, codeword U( j)
is assigned to a bin of codewords which has a bin-index A
j
.
Also generate a second set of |X|
n
i.i.d. bin-indices
˜
A
j
, j = 1, . . . , |X|
n
, according to the uniform pmf over the

set {1,
. . . , m
x
}, independent of (X, Y, Z) and {U( j), A
j
, j =
1, . . . , m
0
u
}. The tuple of bin-indices
e
A := (
˜
A
1
, . . . ,
˜
A
|X|
n
) is
available at locations a and c.
The interpretation is that for j = 1, . . . , |X|
n
, the sourceword
x( j) ∈ C
x
is assigned to a bin of sourcewords which has a
bin-index
˜
A
j
.
Encoding at location-a: Let
J
u
:= min{ j = 1, . . . , m
0
u
: (X, U( j)) ∈ T
(n)
(p
X,U
)}
with the convention that min
j
{} = 1, where {} denotes the
empty set, and where T
(n)
(p
X,U
) denotes the strongly p
X,U
-
typical subset of X
n
× U
n
[1]. Also let J
x
be the index of
X in the tuple of sourcewords C
x
, that is, X = x(J
x
). Set
W
a
:= (A
J
u
,
˜
A
J
x
).
The interpretation is that the encoder at location-a first
“quantizes” the sourceword X to the codeword U(J
u
). This
“quantization” is accomplished by searching through the tuple
of codewords C
u
and finding the first codeword U(J
u
) which
is strongly p
X,U
typical with X. The encoder also randomly
partitions the tuple of codewords C
u
into nonoverlapping bins.
The “quantized” codeword U(J
u
) is assigned to a bin with
bin-index A
J
u
in this random partitioning. In addition, the
encoder also randomly partitions the tuple of all sourcewords
C
x
into nonoverlapping bins. The observed sourceword X,
which occurs at position J
x
in the tuple C
x
, is assigned to a bin
with bin-index
˜
A
J
x
in this random partitioning. The bin-index
A
J
u
of U(J
u
) together with the bin-index
˜
A
J
x
of X = x(J
x
)
comprise the message W
a
sent from location-a to location-b.
Random codebook generation for location-b: Generate m
0
v
i.i.d. relay-codewords V( j) := V
n
( j), j = 1, . . . , m
0
v
, of block-
length n, according to the product pmf p
V
(v) :=
Q
n
i=1
p
V
(v
i
),
independent of (X, Y, Z), {U( j), A
j
, j = 1, . . . , m
0
u
}, and
{
˜
A
j
, j = 1, . . . , |X|
n
}. The tuple of relay-codewords C
v
:=
(V(1), . . . , V(m
0
v
)) is available at locations b and c. Generate
m
0
v
i.i.d. bin-indices B
j
, j = 1, . . . , m
0
v
, according to the
uniform pmf over the set {1, . . . , m
v
}, independent of (X, Y, Z),
{U( j), A
j
, j = 1, . . . , m
0
u
}, {
˜
A
j
, j = 1, . . . , |X|
n
}, and {V( j), j =
1, . . . , m
0
v
}. The tuple of bin-indices B := (B
1
, . . . , B
m
0
v
) is
available at locations b and c.
The interpretation is that for j = 1, . . . , m
0
v
, codeword V( j)
is assigned to a bin of codewords which has a bin-index B
j
.
Encoding at location-b: Noting that W
a
= (A
J
u
,
˜
A
J
x
) and Y
are available at location-b, let
ˆ
J
u
:= min{ j = 1, . . . , m
0
u
: A
j
= A
J
u
, (U( j), Y) ∈ T
(n)
(p
U,Y
)}
and
J
v
:= min{ j = 1, . . . , m
0
v
: (U(
ˆ
J
u
), Y, V( j)) ∈ T
(n)
(p
U,Y,V
)}.
Set W
b
:= (B
J
v
,
˜
A
J
x
).
The interpretation is that the encoder at location-b first esti-
mates the codeword U(J
u
) as U(
ˆ
J
u
). This is accomplished by
searching through the bin of codewords in C
u
whose bin-index
is A
J
u
and finding the first codeword U(
ˆ
J
u
) which is strongly
p
U,Y
typical with Y. The encoder next “jointly-quantizes”
the pair (U(
ˆ
J
u
), Y) to the relay-codeword V(J
v
). This “joint-
quantization” is accomplished by searching through the tuple
of relay-codewords C
v
and finding the first relay-codeword
V(J
v
) which is strongly p
U,Y,V
typical with U(
ˆ
J
u
) and Y. The
encoder also randomly partitions the tuple of relay-codewords
C
v
into nonoverlapping bins. The “quantized” relay-codeword
V(J
v
) is assigned to a bin with bin-index B
J
v
in this random
partitioning. The bin-index B
J
v
of V(J
v
) together with the bin-
index
˜
A
J
x
of X = x(J
x
) comprise the message W
b
sent from
location-b to location-c.
Decoding at location-c: Noting that W
b
= (B
J
v
,
˜
A
J
x
) and Z
are available at location-c, let
ˆ
J
v
:= min{ j = 1, . . . , m
0
v
: B
j
= B
J
v
, (V( j), Z) ∈ T
(n)
(p
V,Z
)}
and
ˆ
J
x
:= min
n
j = 1, . . . , |X|
n
:
˜
A
j
=
˜
A
J
x
, (X( j), V(
ˆ
J
v
), Z) ∈
T
(n)
(p
X,V,Z
)
o
.
Set
b
X := x(
ˆ
J
x
).
The interpretation is that the decoder at location-c first esti-
mates the relay-codeword V(J
v
) as V(
ˆ
J
v
). This is accomplished
by searching through the bin of relay-codewords in C
v
whose
bin-index is B
J
v
and finding the first codeword V(
ˆ
J
v
) which
is strongly p
V,Z
typical with Z. The decoder next estimates
the sourceword X = x(J
x
) as
b
X = x(
ˆ
J
x
). This is accomplished
by searching through the set of all sourcewords in C
x
whose
bin-index is
˜
A
J
x
and finding the first sourceword X(
ˆ
J
x
) which
is strongly p
X,V,Z
typical with V(
ˆ
J
v
) and Z.
Error events:
E
1
: {X < T
(n)
(p
X
)}.
As n ↑ ∞, by the strong law of larger numbers, P(E
1
) ↓ 0.
E
2
: {(X, U(J
u
)) < T
(n)
(p
X,U
)}.
There exists an
1
(n, ) > 0 such that (i)
1
(n, ) ↓ 0 as n ↑ ∞
and
↓
0 and (ii)
P
(
E
2
| E
c
1
)
↓
0 if
(1/n) log
2
m
0
u
= I(X; U) +
1
(n, ). (4.9)
E
3
: {(X, U(J
u
), Y) < T
(n)
(p
X,U,Y
)}.
P(E
3
| E
c
2
) ↓ 0 as n ↑ ∞ by the Markov lemma [10] because
U–X–Y is a Markov chain.
E
4
: {∃ j , J
u
: A
j
= A
J
u
, (U( j), Y) ∈ T
(n)
(p
U,Y
)},
There exists an
2
(n, ) > 0 such that (i)
2
(n, ) ↓ 0 as n ↑ ∞
and ↓ 0 and (ii) P(E
4
) ↓ 0 if
(1/n)(log
2
m
0
u
− log
2
m
u
) = I(U; Y) −
2
(n, ). (4.10)
Conditions (4.9) and (4.10) and the Markov chain U–X–(Y, Z)
imply the following condition on (1/n) log
2
m
u
:
1
n
log
2
m
u
−
1
(n,
) −
2
(n, ) = I(X; U) − I(U; Y)
= I(X; U|Y). (4.11)
Hence under condition (4.11), P(
ˆ
J
u
, J
u
) ↓ 0 as n ↑ ∞.
E
5
: {(U(J
u
), Y, V(J
v
)) < T
(n)
(p
X,U,Y,V
)},
There exists an
3
(n, ) > 0 such that (i)
3
(n, ) ↓ 0 as n ↑ ∞
and ↓ 0 and (ii) P(E
5
| (E
3
∪ E
4
)
c
) ↓ 0 if
(1/n) log
2
m
0
v
= I(U, Y; V) +
3
(n, ). (4.12)