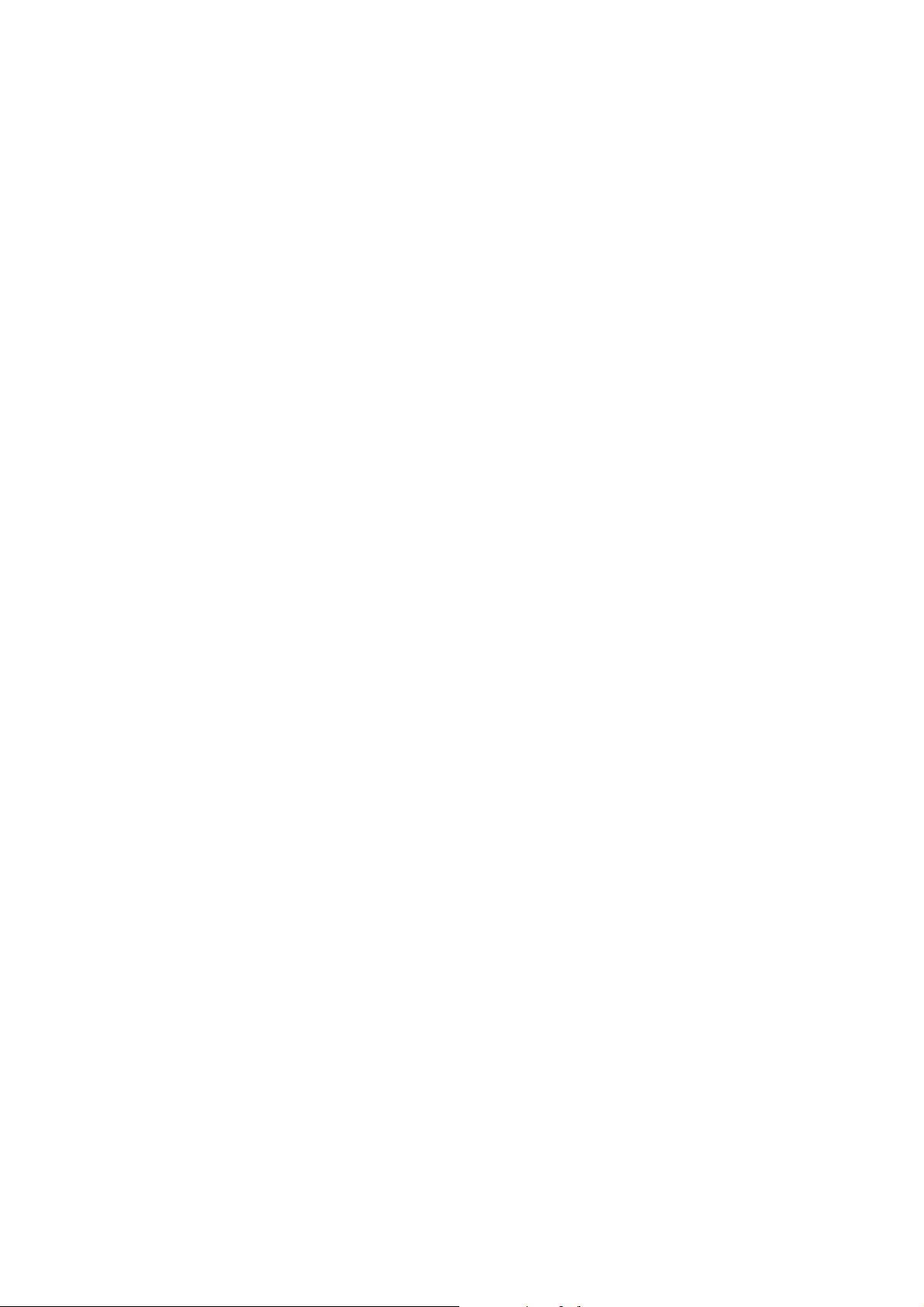




read more
The forward feature selection method is used to find low dimensional subsets of category-specific features by predominately selecting features, which occur almost exclusively for a certain category.
Due to the fact that the objects are presented by hand, skin color parts in the segment are systematic noise, which the authors remove from the initial foreground hypothesis based on the detection method proposed by Fritsch et al. (2002).
The major drawback of those architectures commonly used for identification tasks is the inefficient separation of cooccuring categories.
It seems to be that for their categorization task the indepen-dent representation of categories somehow weakens the forgetting effect of SLP networks.
A common strategy for life-long learning architectures e.g. (Hamker, 2001; Furao & Hasegawa, 2006; Kirstein et al., 2008) is the usage of a node specific learning rate combined with an incremental node insertion rule.
The advantages of these approaches are their robustness against partial occlusion, scale changes, and the ability to deal with cluttered environments.
For color categories the effect of imprecise foreground masks on the categorization performance seems also to be only minor, otherwise the performance would be considerably lower.
This allows that object views can be first used to test the STM and LTM representation and after providing confirmed labels the same views can also be used to enhance the representation by transferring them into the STM, even if they where recorded before the confirmation.
Based on the currently available feature vectors, the learning methods are used to incorporate this STM knowledge into the LTM by applying the learning dynamics of the cLVQ method described in Section 4.2.3.
To relax this separation and to make the most efficient use of object views, the authors introduce a sensory memory concept for temporarily remembering views of the currently attended object, by using the same one-shot learning method as used for the STM.
(1)This computation of local edge responses is restricted to the positions in the foreground mask with ξi(x, y) > 0, whereas the ∗ denotes the inner product of two vectors.
One of the essential problems when dealing with learning in unconstrained environments is the definition of a shared attention concept between the learning system and the human tutor.
Additionally the hypothesis list is repeatedly communicated to the user (in 5 second intervals), while newly acquired segments are also used to refine this list.
Additionally this constraint strongly reduces the appearance variations of the presented objects and therefore makes the category learning task much easier.
The authors could show that their learning system can efficiently perform all necessary processing steps including figure-ground segregation, feature extraction and incremental learning.