
A Comparison of Random Walks in
Dependent Random Environments
Werner R.W. Scheinhardt
University of Twente
w.r.w.scheinhardt@utwente.nl
Dirk P. Kroese
The University of Queensland
kroese@maths.uq.edu.au
April 13, 2015
Abstract
We provide exact computations for the drift of random walks in depen-
dent random environments, including k-dependent and moving average envi-
ronments. We show how the drift can be characterized and evaluated using
Perron-Frobenius theory. Comparing random walks in various dependent en-
vironments, we demonstrate that their drifts can exhibit interesting behavior
that depends significantly on the dependency structure of the random envi-
ronment.
MSC subject classifications: Primary 60K37, 60G50; secondary 82B41
Keywords: random walk, depen dent random environment, drift, Perron–
Frobenius eigenvalue
1 Introduction
Random walks in random environments (RWREs) are well-known mathematical
models for motion through disorganized (random) media. They generalize ordinary
random walks whereby the transition probabilities from any position are determined
by the random state of the environment at that position. RWREs exhibit interesting
and unusual behavior that is not seen in ordinary random walks. For example, the
walk can tend to infinity almost surely, while its overall drift is 0. The reason for
such surprising behavior is that RWREs can spend a long time in (rare) re gions
from which it is difficult to escape — in effect, the walker becomes “trapped” for a
long time.
Since the late 1960s a vast body of knowledge has been built up on the behav-
ior of RWREs. Early applications can be found in Chernov [
4] and Temkin [17];
see also Kozlov [
9] and references therein. Recent applications to charge transport
in designed materials are given in Brereton et al. [3] and Stenze l et al. [15]. The
mathematical framework for one-dimensional RWREs in independent environments
was laid by So lomon [14], and was further ex tended by K esten et al. [8], Sinai [13],
1

Greven and Den Hollander [6]. Markovian environments were investigated in Dol-
gopyat [5] and Mayer-Wolf et al. [10]. Alili [1] showed that in the one-dimensional
case much of the theory for independent env ironments could be generalized to the
case where the environment process is stationary and ergodic. Overviews of the
current state of the art, with a foc us on higher-dimensional RWREs, can be found,
for example, in Hughes [
7], Sznitman [16], Zeitouni [18, 19], and R´ev´esz [1 1].
Although from a theoretical perspective the behavior of one-dimensional RWREs
is well understood, from an applied and computational point of view significant
gaps in our understanding remain. For e xample, exact drift computatio ns and com-
parisons (as oposed to comparisons using simulation) be twee n dependent random
environments seem to be entirely missing from the literature. The reason is that
such exact computations are not trivial and require additional insights.
The contribution of this paper is twofold. First, we provide new methodology
and explicit expressions for the c omputation of the drift of one-dimensiona l random
walks in various dependent environments, focusing on so-called ‘swap models’. In
particular, our approach is based on Perron–Frobenius theory, which allows ea sy
computation of the drift and as well a s various cutoff points for transient/recurrent
behavior. Second, we compare the drift behavior between various dependent envi-
ronments, including moving avera ge and k-dependent e nvironments. We show that
this behavior c an deviate considerably from that of the (known) independent c ase.
The rest of the pape r is organized as follows. In Section
2 we formulate the
model for a one-dimensional RWRE in a stationary and ergodic environment and
review some of the key results fro m [
1]. We then formulate a flexible mechanism
for constructing depe ndent random environment that includes the iid, Markovian,
k-dependent, and moving average environments. In Section
3 we prove explicit
(computable) results for the drift for each of these models, and compare their be-
haviors. Conclusions and directions for future research are given in Section
4.
2 Model and preliminaries
In this section we review so me key results on one-dimens ional RWREs and introduce
the class of ‘swap-models’ that we will study in more detail.
2.1 General theory
Consider a stochastic process {X
n
, n = 0, 1, 2, . . .} with state space Z, and a sto chas-
tic “Underlying” environment U taking value s in some set U
Z
, where U is the set
of possible environment states for each site in Z. We assume that U is statio nary
2

(under P) as well as ergodic (under the natural shift operator on Z). The e volution
of {X
n
} depends on the rea liz ation of U, which is random but fixed in time. For
any re alization u of U the process {X
n
} behaves a s a simple random walk with
transition probabilities
P(X
n+1
= i + 1 | X
n
= i, U = u) = α
i
(u)
P(X
n+1
= i − 1 | X
n
= i, U = u) = β
i
(u) = 1 − α
i
(u).
(2.1)
The theor e tical behavior of {X
n
} is well understood, as set out in the seminal
work of Solomon [
14]. In particular, Theorems 2.1 and 2.2 below c ompletely de-
scribe the transience/recurrence behavior and the Law of Large Numbers behavior
of {X
n
}. We follow the notation of Alili [
1] and first give the key q uantities that
appear in these theorems. Define
σ
i
= σ
i
(u) =
β
i
(u)
α
i
(u)
, i ∈ Z , (2.2)
and let
S = 1 + σ
1
+ σ
1
σ
2
+ σ
1
σ
2
σ
3
+ · · · (2.3)
and
F = 1 +
1
σ
−1
+
1
σ
−1
σ
−2
+
1
σ
−1
σ
−2
σ
−3
+ · · · (2.4)
Theorem 2.1. (Theorem 2.1 in [
1])
1. If E[ln σ
0
] < 0, then almost surely lim
n→∞
X
n
= ∞ .
2. If E[ln σ
0
] > 0, then almost surely lim
n→∞
X
n
= −∞ .
3. If E[ln σ
0
] = 0, then almost surely lim inf
n→∞
X
n
= −∞ and lim sup
n→∞
X
n
= ∞ .
Theorem 2.2. (Theorem 4.1 in [1])
1. If E[S] < ∞, then almost surely lim
n→∞
X
n
n
=
1
E[(1 + σ
0
)S]
=
1
2E[S] − 1
.
2. If E[F ] < ∞, then almost surely lim
n→∞
X
n
n
=
−1
E[(1 + σ
−1
0
)F ]
=
−1
2E[F ] − 1
.
3. If E[S] = ∞ and E[F ] = ∞, then almost surely lim
n→∞
X
n
n
= 0.
Note that we have added the second equalities in statements 1. and 2. of Theo-
rem
2.2. These follow directly from the stationarity of U.
We will call lim
n→∞
X
n
/n the drift of the proce ss {X
n
}, and denote it by V .
Note that, as mentioned in the introduction, it is possible for the chain to be
transient with drift 0 (namely when E[ln σ
0
] 6= 0, E[S] = ∞ and E[F] = ∞).
3
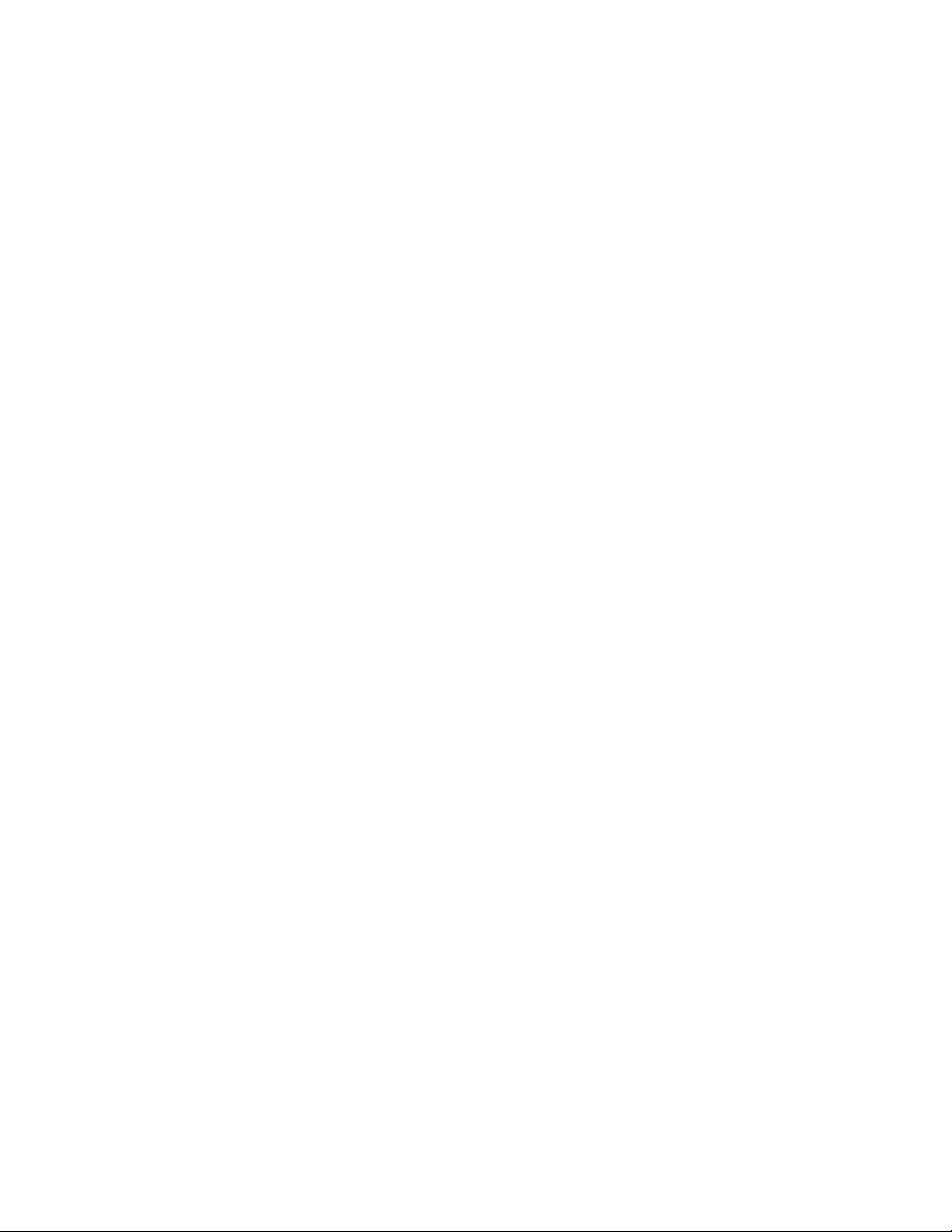
2.2 Swap model
We next focus on what we will c all swap models, as studied by Sinai [
13]. Here,
U = {−1, 1}; that is , we assume that all elements U
i
of the process U ta ke value
either −1 or +1. We assume that the transition probabilities in state i only depends
on U
i
and not on other e lements of U, as follows. When U
i
= −1, the transition
probabilities of {X
n
} fr om state i to states i + 1 a nd i − 1 are swapped with r esp ect
to the values they have when U
i
= + 1. Thus, for some fixed value p in (0, 1) we
let α
i
(u) = p (and β
i
(u) = 1 − p) if u
i
= 1, and α
i
(u) = 1 − p (and β
i
(u) = p) if
u
i
= −1. Thus, (2.1) becomes
P(X
n+1
= i + 1 | X
n
= i, U = u) =
(
p if u
i
= 1
1 − p if u
i
= −1
and
P(X
n+1
= i − 1 | X
n
= i, U = u) =
(
1 − p if u
i
= 1
p if u
i
= −1 .
Next, we choose a dependence structure fo r U using the following s imple, but
novel, construction. Let {Y
i
, i ∈ Z} be a sta tionary and ergodic Markov chain
taking values in some finite set M and let g : M → {−1, 1} be a given function.
Now define the environment at state i as U
i
= g(Y
i
), i ∈ Z. Despite its simplicity,
this formalism covers a number of interesting dependence structures on U, discussed
next.
iid environment. In this case the {U
i
} are i.i.d. random variables, with α
def
=
P(U
i
= 1 ) = 1 − P(U
i
= − 1). Formally, this fits the framework above by choosing
g the identity function on M = {−1, 1} and {Y
i
} the Markov chain with one-step
transition probabilities P(Y
i
= 1 | Y
i−1
= −1) = P(Y
i
= 1 | Y
i−1
= 1) = α for all i.
k-dependent environment. Define a k-dependent environment as an environ-
ment {U
i
} for which
P(U
i
= u
i
| U
i−1
= u
i−1
, U
i−2
= u
i−2
, . . .) = (2.5)
P(U
i
= u
i
| U
i−1
= u
i−1
, U
i−2
= u
i−2
, . . . , U
i−k+1
= u
i−k+1
), u
j
∈ {− 1, 1}. (2.6)
Spec ial cases are the independent environment (k = 0; see above) and the so-called
Markovian environment (k = 1). For k > 1, let {Y
i
, i ∈ Z} be a Markov chain
that takes values in M = {−1, 1}
k
such that from any state (u
i−k
, . . . , u
i−1
) only
two possible transitions can ta ke place, given by
(u
i−k
, . . . , u
i−1
) → (u
i−k+1
, . . . , u
i−1
, u
i
), u
i
∈ {− 1, 1},
4

with corresponding probabilities 1−a
(u
i−k
,...,u
i−2
)
, a
(u
i−k
,...,u
i−2
)
, b
(u
i−k
,...,u
i−2
)
, and
1 − b
(u
i−k
,...,u
i−2
)
, for (u
i−1
, u
i
) equal to (−1, −1), (−1, 1), (1, −1), and (1, 1), re-
spectively. Now define U
i
as the last component of Y
i
. Then {U
i
, i ∈ Z} is a
k-dependent environment, and Y
i
= (U
i−k+1
, . . . , U
i
). In the special case k = 1
(Markovian environment), we omit the subindices of a (transition probability from
U
i−1
= −1 to U
i
= +1) and b (from U
i−1
= +1 to U
i
= −1).
Moving average environment. Cons ide r a “ moving average” environment, which
is built up in two phases as follows. First, start with an iid environment {
b
U
i
} as
in the iid case, with P(
b
U
i
= 1) = α. Let Y
i
= (
b
U
i
,
b
U
i+1
,
b
U
i+2
). Hence, {Y
i
} is
a Markov process with states 1 = (−1, −1, −1), 2 = (−1, −1, 1), . . . , 8 = (1, 1, 1)
(lexicographical o rder). The corresponding transition matrix is given by
P =
1 − α α 0 0 0 0 0 0
0 0 1 − α α 0 0 0 0
0 0 0 0 1 − α α 0 0
0 0 0 0 0 0 1 − α α
1 − α α 0 0 0 0 0 0
0 0 1 − α α 0 0 0 0
0 0 0 0 1 − α α 0 0
0 0 0 0 0 0 1 − α α
. (2.7)
Now define U
i
= g(Y
i
), where g(Y
i
) = 1 if at le ast two of the three random var iables
b
U
i
,
b
U
i+1
and
b
U
i+2
are 1, and g(Y
i
) = −1 otherwise. Thus,
(g(1), . . . , g(8)) = (−1, −1, −1, 1, −1, 1, 1, 1) , (2.8)
and we see that ea ch U
i
is obtained by taking the moving average of
b
U
i
,
b
U
i+1
and
b
U
i+2
, as illustrated in Fig ure 2.2.
Figure 1: Moving average environment.
3 Evaluating the drift
In this section we first give the gene ral solution approach for the Markov-based
swap model, and then further specify the transience/recurrence and drift results to
the Markov environment, the 2-dependent environment, and the moving average
environments. We omit a separate derivation for the i.i.d. environment, which can
be viewed as a special case of the Markovian environment, see Remark
3.1.
5