
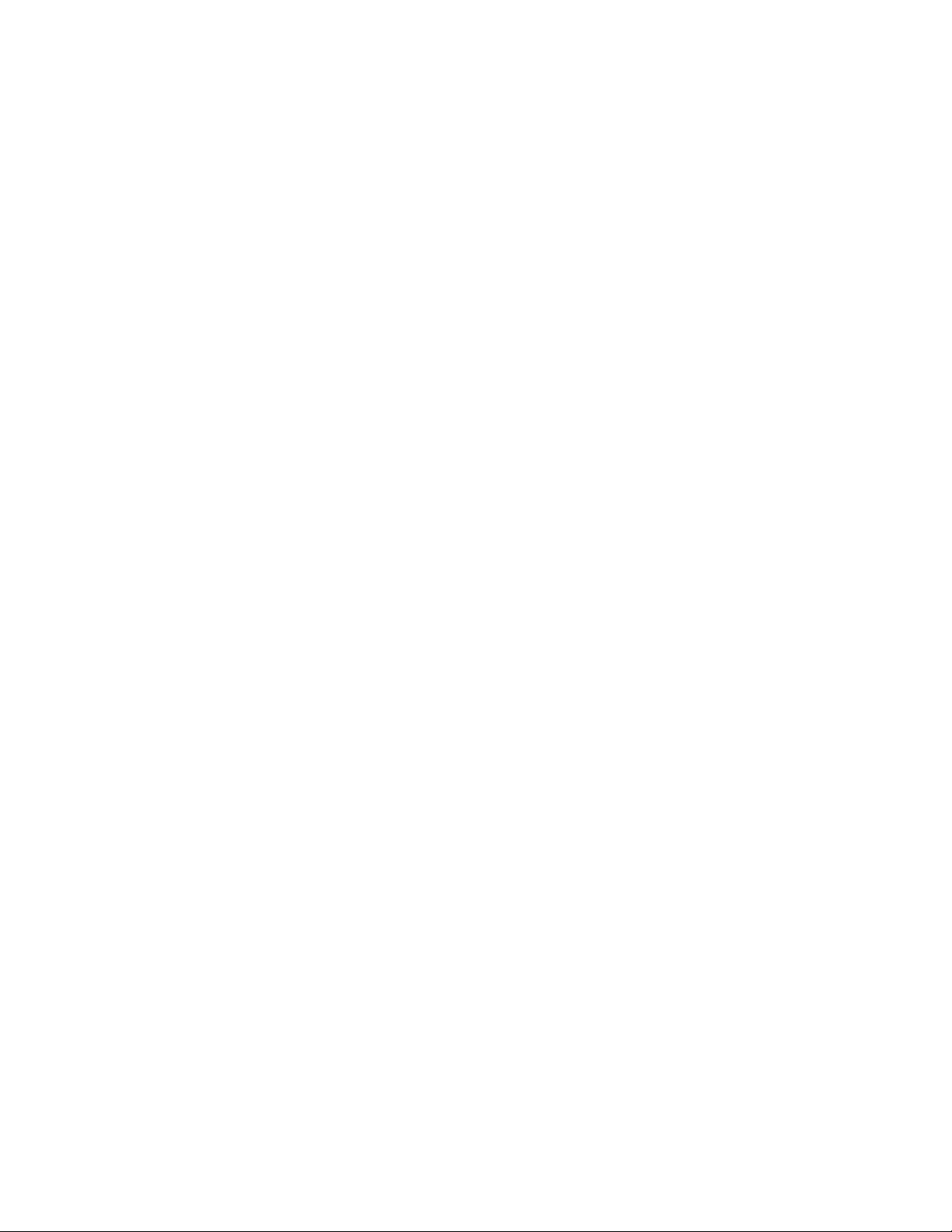

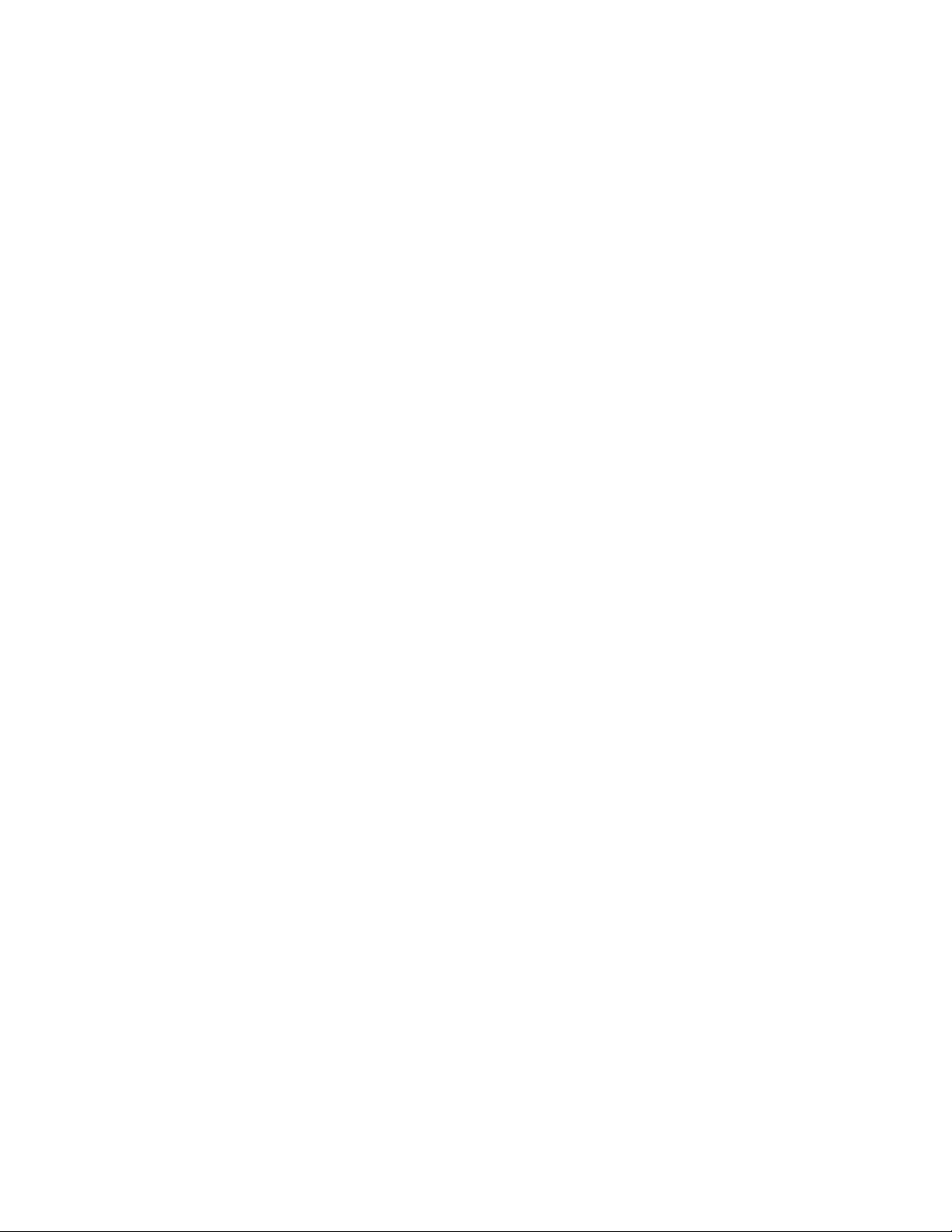

read more
In the future, the authors plan to generate patches for new types of bug reports, and extend R2Fix to take the output of existing bug detection tools as input to improve the effectiveness of patch generation. In other words, R2Fix does not use the “ Comment ” fields of the bug reports, because the authors want to apply R2Fix as soon as a bug is reported to maximize the time and effort that R2Fix can save for developers in fixing bugs. The authors estimate that it will take you approximately 3 minutes to complete this short survey. In other words, R2Fix does not use the “ Comment ” fields of the bug reports, because the authors want to apply R2Fix as soon as a bug is reported to maximize the time and effort that R2Fix can save for developers in fixing bugs.
The patch deletes the line that writes 5 bytes to buffer state (denoted by - strcpy(state, "off ");), and adds a new line to write only 4 bytes to state (+ strcpy(state, "off");), which fixes the overflow bug.
The developers first need to understand this bug report by reading the relevant code together with this report: the buffer state contains only 4 bytes, but 5 bytes, “off \\0”, was written to the buffer, where denotes one space character and the single character ‘\\0’ is needed to mark the end of the string.
My dear mother, the first teacher and the role model in my life, gives me confidence to explore new things, especially in a different country far away from my homeland.
Developers’ bug-fixing process is primarily manual; therefore the time required for producing a fix and its accuracy depend on the skill and experience of individuals.
Developers often spend days, weeks, or even months diagnosing the root cause of a bug by reading the relevant source code, using a debugger to observe and modify the program execution on different inputs, etc.
After a developer determines the root cause, typically the developer needs to figure out how to modify the buggy code to fix the bug, check out the buggy version of the software, apply the fix, and generate the patch.
I am thankful to readers of the thesis, Prof. Patrick Lam and Prof. Mahesh V. Tripunitara, for spending their valuable time to review the thesis and give valuable comments.